

What on earth is R actually doing?While Rs built in function do work, were going to introduce you to another method for repeating things using the package plyr. In both cases there are three addition operations to perform. Try to Why on earth should these take a different amount of time to calculate? Linear algebra isn’t magic. In question 3 you generated a loop to go over a data frame. Write a loop that loops over the columns and reports the mean of the column if it is numeric and the total number of characters if it’s a character vector.
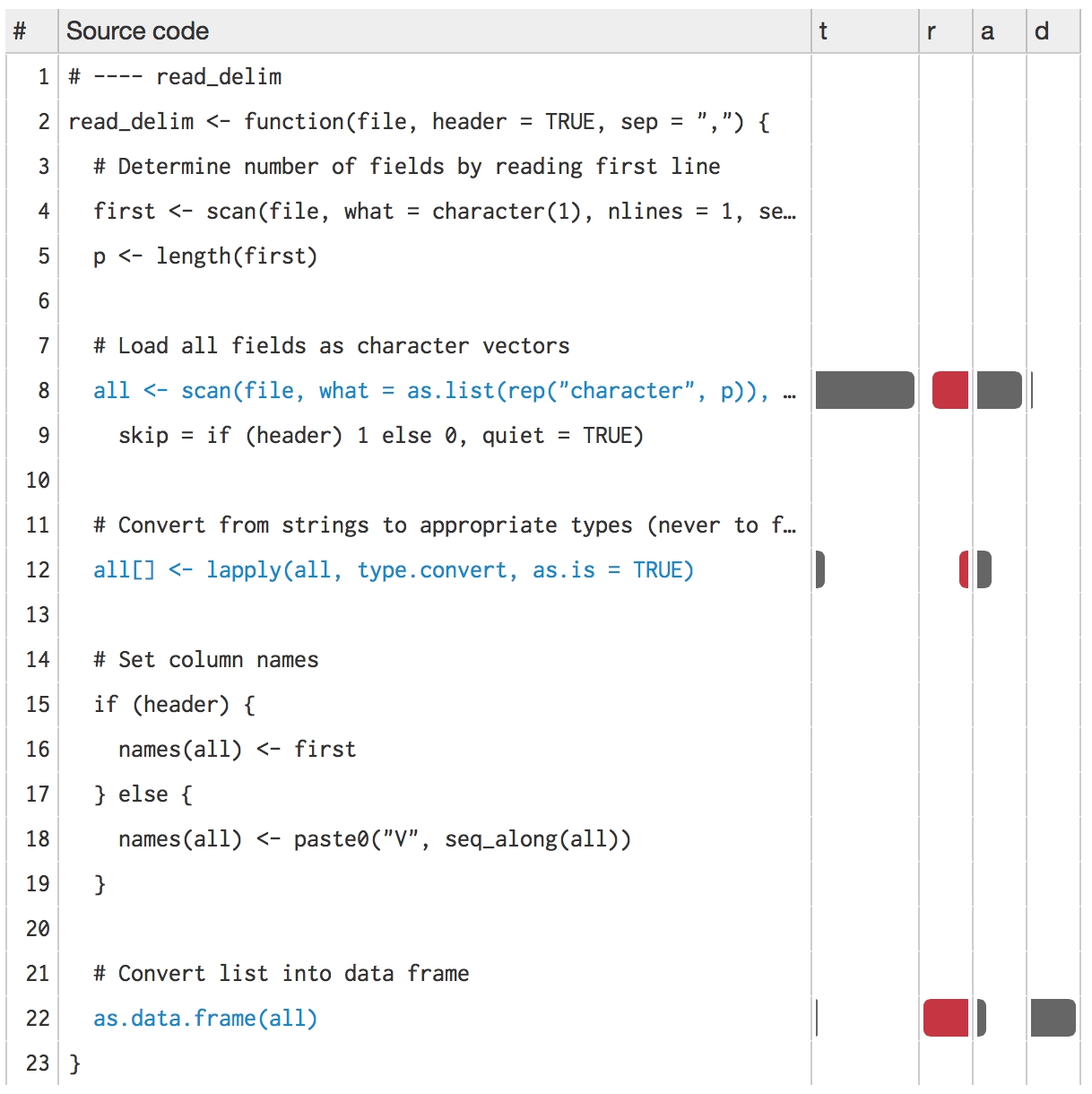
Writing A Loop In R Code That You
The loops in a vectorised function are written in C instead of R. Writing code that you think is faster, but is actually no better. The while loop, set in the middle of the figure above, is made of an initialization block as before. The next sections will take a closer look at each of these structures that are shown in the figure above.
These means R is calling a C, C++, or FORTRAN program to carry out operations. If you look at their source code, it will include. You’ll see this in many R functions.
If you do the latter, R has to do the “figuring out” stuff, as well as the translation, each time. The compiled code is able to run faster than code written in pure R, because the “figuring out” stuff is done first, and it can zoom ahead without the “translation” steps that R needs.If you need to run a function over all the values in a vector, you could pass a whole vector through the R function to the compiled code, or you could call the R function repeatedly for each value. In fft() the compiled code runs only after R figures out the data type in z, and also whether to use the default value of inverse.
Despite all of its flexibility, R does have some restrictions on what we can do. Since this occurs in the compiled code, though, without the overhead of R functions, this is much faster.Another important component of the speed of vectorized operations is that vectors in R are typed. This is inevitable somehow the computer is going to need to operate on each element of your vector.
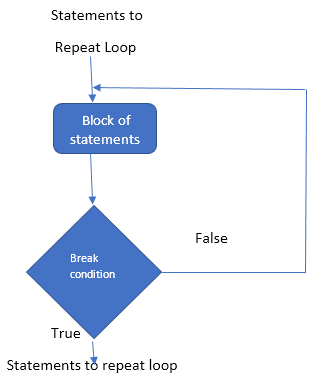
In other languages, short vectors might be better expressed as scalars. There’s no advantage to NOT organizing your data as vector. You’re welcome.This means that, in R, typing “6” tells R something like 6While in other languages, “6” might just be 6So, while in other languages, it might be more efficient to express something as a single number rather than a length-one vector, in R this is impossible. To quote Tim Smith in “aRrgh: a newcomer’s (angry) guide to R”All naked numbers are double-width floating-point atomic vectors of length one. Everything is a vectorIn R everything is a vector.
For certain problems, a shiny new BLAS can considerably speed up code, but results vary depending on the specific linear algebra operations you are using. This used to be like putting a new engine in your car, but it’s gotten considerably easier. So if your calculations can be expressed in actual linear algebra terms, such as matrix multiplication, than it is almost certainly faster to vectorize them because the BLAS will be doing most of the heavy lifting.There are faster and slower linear algebra libraries, and you can install new ones on your computer and tell R to use them instead of the defaults. A BLAS is generally designed to be highly efficient and has things like built-in parallel processing, hardware-specific implementation, and a host of other tricks. R, and a lot of other software, relies on these specialized programs and outsources linear algebra to them. Such a program is called a BLAS - basic linear algebra system.
It has to find the vector in memory, create a new vector that will fit more data, copy the old data over, insert the new data, and erase the old vector. So one of the slower ways to write a for loop is to resize a vector repeatedly, so that R has to re-allocate memory repeatedly, like this: j <- 1Here, in each repetition of the for loop, R has to re-size the vector and re-allocate memory. Functionals mostly are written in pure R, and they speed up code only in certain cases.One operation that is slow in R, and somewhat slow in all languages, is memory allocation. Because these can use arbitrary functions, they are NOT compiled.
This is considered bad practice sometimes. In a for loop, on the other hand, when you do something like for(i in 1:10), you get the leftover i in your environment. When you run a ply function, everything happens inside that function, and nothing changes in your working environment (this is known as “functional programming”). This is the main reason that they can be faster.Another thing that “ply” functions help with is avoiding what are known as side effects. Here’s how you’d do that for the above case: j <- rep(NA, 10)The apply or plyr::*ply functions all actually have for loops inside, but they automatically do things like pre-allocating vector size so you don’t screw it up.
For instance, here is a good example of implementing a random walk using vectorized code. In some cases where the obvious implementation of an algorithm uses a for loop, there’s a vectorized way around it. Loops where each iteration is dependent on the results of previous iterationsNote that the second case is tricky. Using functions that don’t take vector arguments So when might for loops make sense over vectorization?There are still situations that it may make sense to use for loops instead of vectorized functions, though. Once you are used to writing vectorized code in general, though, for loops in R will can seem odd.
Good discussion in a couple of blog posts by John Myles White. Some resources on vectorization It may make sense to use a for loop in such cases, especially if they are more intuitive or easier to read for you. In these cases, looping and overhead from function calls make up a small fraction of your computational time. Examples of such functions include cumsum (cumulative sums), rle (counting number of repeated value), and ifelse (vectorized if…else statements).Your performance penalty for using a for loop instead a vector will be small if the number of iterations is relatively small, and the functions called inside your for loop are slow.
